Dr Matt Ellis
Department of Computer Science
Lecturer in Machine Learning
Member of the Machine Learning research group
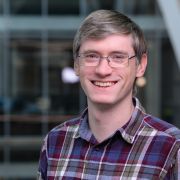
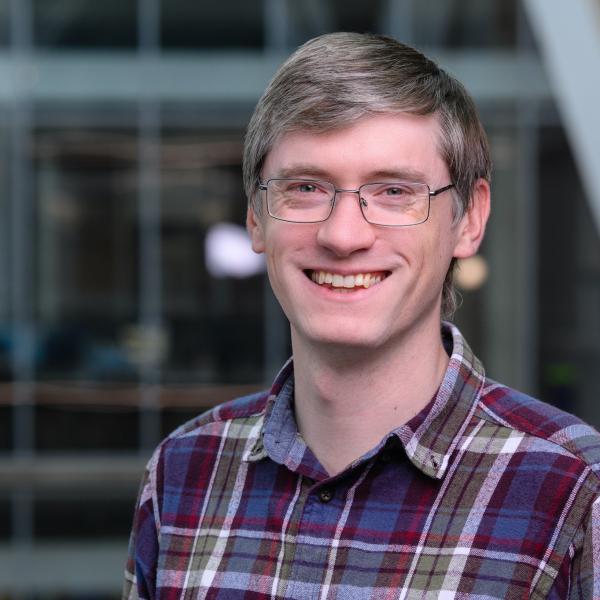
+44 114 222 1949
Full contact details
Department of Computer Science
Regent Court (DCS)
211 Portobello
Sheffield
S1 4DP
- Profile
-
Dr Matthew Ellis is a Lecturer in Machine Learning and member of the Machine Learning Group at the Department of Computer Science.
He graduated with a MPhys in Theoretical Physics from the University of York in 2011, before staying at York to undertake a PhD in Physics under Prof. Roy Chantrell.
After completing his PhD in 2015 he joined the group of Prof. Stefano Sanvito at Trinity College Dublin as a post-doctoral research fellow. In 2019, he joined the University of Sheffield as a post-doctoral research associate in the Bio-Inpsired Machine Learning group under Prof. Eleni Vasilaki developing machine learning models for neuromorphic computing in collaboration with the Department of Materials Science.
- Research interests
-
Dr Ellis is interested in developing energy efficient machine learning algorithms and systems based on neuromorphic computing. In particular, he is interested in developing models of physical systems that can be utilised as machine learning processing devices, such as devices for physical reservoir computing or neuromorphic hardware based on magnetic systems. Beyond machine learning he is interested in developing large scale models of magnetic devices including developing gpu accelerated models.
- Publications
-
Show: Featured publications All publications
Featured publications
Journal articles
- Atomistic spin model simulations of magnetic nanomaterials. Journal of Physics: Condensed Matter, 26(10), 103202-103202.
All publications
Journal articles
- A perspective on physical reservoir computing with nanomagnetic devices. Applied Physics Letters, 122(4), 040501-040501.
- Neuromorphic computation with a single magnetic domain wall. Scientific Reports, 11(1). View this article in WRRO
- Voltage-controlled superparamagnetic ensembles for low-power reservoir computing. Applied Physics Letters, 118(20).
- Dynamically‐driven emergence in a nanomagnetic system. Advanced Functional Materials. View this article in WRRO
- Role of longitudinal fluctuations in L10 FePt. Physical Review B, 100(21). View this article in WRRO
- Nontrivial spatial dependence of the spin torques in L10 FePt-based tunneling junctions. Physical Review B, 99(1). View this article in WRRO
- Anomalous damping dependence of the switching time in Fe/FePt bilayer recording media. Physical Review B, 99(2). View this article in WRRO
- Multiscale modeling of current-induced switching in magnetic tunnel junctions using ab initio spin-transfer torques. Physical Review B, 96(22).
- Manifestation of higher-order inter-granular exchange in magnetic recording media. Applied Physics Letters, 111(8).
- All-optical switching in granular ferromagnets caused by magnetic circular dichroism. Scientific Reports, 6. View this article in WRRO
- Switching times of nanoscale FePt: Finite size effects on the linear reversal mechanism. Applied Physics Letters, 106(16). View this article in WRRO
- The Landau–Lifshitz equation in atomistic models. Low Temperature Physics, 41(9), 705-712.
- Atomistic spin model simulations of magnetic nanomaterials. Journal of Physics: Condensed Matter, 26(10), 103202-103202.
- Quantifying the Computational Capability of a Nanomagnetic Reservoir Computing Platform with Emergent Magnetisation Dynamics. Nanotechnology.
- Temperature-dependent ferromagnetic resonance via the Landau-Lifshitz-Bloch equation: Application to FePt. Physical Review B, 90(9).
- Classical spin model of the relaxation dynamics of rare-earth doped permalloy. Physical Review B, 86(17).
- View this article in WRRO
- Spin-lattice dynamics model with angular momentum transfer for canonical and microcanonical ensembles. Physical Review B, 103(2).
Conference proceedings papers
- From stochasticity to functionality: harnessing magnetic domain walls for probabilistic and neuromorphic computing. Spintronics XIV, 1 August 2021 - 5 August 2021.
Preprints
- Neuromorphic Computation With a Single Magnetic Domain Wall.
- Atomistic spin model simulations of magnetic nanomaterials. Journal of Physics: Condensed Matter, 26(10), 103202-103202.